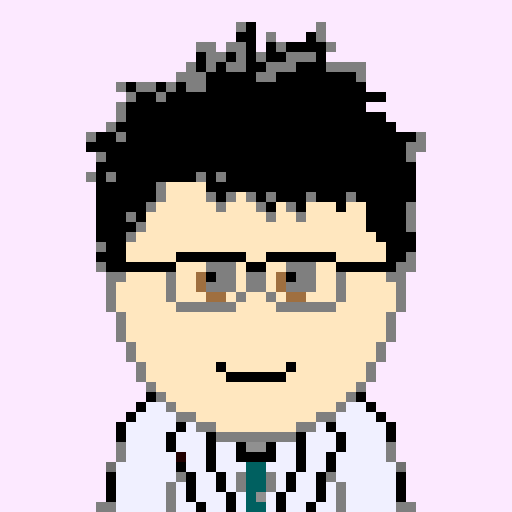
前回・前々回と違い、テキストデータの保存について。
こちらは、Word IndexをWordに変換する必要があります。
はじめに
Keras のload_dataで読み込んだデータを可視化する方法。
(1) CIFAR10、CIFAR100 画像への変換方法
(2) MNIST、Fashin-MNIST 画像への変換方法
(3) IMDB Review、Reuters Topics テキストへの変換方法
(4) Boston Housing data のcsvへの変換方法
この記事では「(3) IMDB Review、Reuters Topics テキストへの変換方法」について書いています。
ソースコード一式
こちらに(1)~(4) すべてのコードを一括したファイルをアップしています。
コード見たほうが早いわーな方はどうぞ。
IMDB Review、Reuters Topicsのテキスト化保存
この2つは、同じ手法で保存できます。
データセットは「int配列(可変長)」であり、
それに対する正解値は「0:Positive」「1:Negative」の2クラスです。
IMDB Reviewのデータ→テキスト化
from tensorflow.keras.datasets import imdb, reuters
# Load data from keras API
(x_train, y_train), (x_test, y_test) = imdb.load_data()
まず、load_data()で、train, testにデータを読み込みます。
y_train, y_testには、この時点で
[0,1,1・・・] のように、0:Positive か 1:Negativeかの正解値が入っています。
x_train, x_testには、この時点で
[[1, 14, 22, 16, 43, 530, 973, 1622・・], [1, ・・・], [1, ・・・]] のように、
可変長のWord Index配列が順番に入っています。
この、1, 14, 22, 16・・・というのが、以下のWord Indexに対応します。
(0~2までは予約語)
Word Index | Word |
0 | (無効) |
1 | (無効) |
2 | (無効) |
3 | |
4 | the |
5 | and |
6 | a |
7 | of |
8 | to |
9 | is |
10 | br |
11 | in |
12 | it |
13 | i |
14 | this |
15 | that |
16 | was |
17 | as |
18 | for |
19 | with |
20 | movie |
21 | but |
22 | film |
23 | on |
24 | not |
・・・ | ・・・ |
などなど・・・全部で9万近くあります。
このWord Indexを用いて復元すると、
1, 14, 24, 16, 43・・というのが、
this film was just brilliant casting location scenery story direction ~~~
のような文章に変換できます。
これらを、テキストファイルにして1つずつ保存していきます。
このWord Indexは imdb.get_word_index() で取得できますが、
そのままの状態では、{‘key’:’value’} の辞書型になっています。
・・・ 'artbox': 88582, 'cronyn': 52004, 'hardboiled': 52005, "voorhees'": 88583, '35mm': 16815, "'l'": 88584, 'paget': 18509, 'expands': 20597}
これを、テキスト化しやすいように、
最初にリストに変換しておきます。
(word_list[word_index] で変換できるよう)
# make dictionary to reference index
word_list = {(value+3):key for key,value in word_index.items()}
INVALID_STR = '#$%'
word_list[0] = INVALID_STR
word_list[1] = INVALID_STR
word_list[2] = INVALID_STR
さらには、
word index:0~2 は予約なので、テキトーな値(#$%)を埋めています。
(後にreplaceで一括で削除するためのダミーデータです)
保存先フォルダを先に生成します。
“0_positive”, “1_negative”の名前で作成します。
この下に、レビューデータをテキスト化して保存していきます。
OUT_DIR = 'IMDB'
# define class names 0:negative / 1:positive
class_list = ['negative','positive']
# make train/test dirs and class dirs
for cid, class_name in enumerate(class_list):
os.makedirs(os.path.join(OUT_DIR,'train', '{:d}_{}'.format(cid,class_name)), exist_ok=True)
os.makedirs(os.path.join(OUT_DIR,'test', '{:d}_{}'.format(cid,class_name)), exist_ok=True)
では、
メインの変換部です。
実際には、
word_org = ‘ ‘.join(word_list[inx] for inx in x_data )
として、1行でfor文を回しながら、スペース区切りでテキスト化しています。
# convert train data
for num, (x_data, y_data) in enumerate(zip(x_train, y_train)):
# make file path
fpath = os.path.join(OUT_DIR,'train', '{:d}_{}'.format(y_data,class_list[y_data]), 'train_{:05d}.txt'.format(num))
with open(fpath, mode='w', encoding='utf-8') as f:
# convert indices and join words with space
word_org = ' '.join(word_list[inx] for inx in x_data )
# remove invalid strings
word_org = word_org.replace(INVALID_STR+' ', '')
# save text
f.write(word_org)
さらに、
最初に埋めておいたダミーデータを削除
word_org = word_org.replace(INVALID_STR+’ ‘, ”)
この後にf.writeで書き込み、保存完了です。
これらをTrain, Testともに処理して終了。
以下のように保存されます。
Reuters Topicsのデータ→テキスト化
こちらも、IMDBとほぼ同じです。
違いは、IMDBが2クラスなのにたいし、カテゴリが46あるので、
そのカテゴリ分のフォルダを作成します。
カテゴリID-カテゴリ名の対応は、以下のサイトなどに記されています。
ID | Category |
0 | cocoa |
1 | grain |
2 | veg-oil |
3 | earn |
4 | acq |
5 | wheat |
6 | copper |
7 | housing |
8 | money-supply |
9 | coffee |
10 | sugar |
11 | trade |
12 | reserves |
13 | ship |
14 | cotton |
15 | carcass |
16 | crude |
17 | nat-gas |
18 | cpi |
19 | money-fx |
20 | interest |
21 | gnp |
22 | meal-feed |
23 | alum |
24 | oilseed |
25 | gold |
26 | tin |
27 | strategic-metal |
28 | livestock |
29 | retail |
30 | ipi |
31 | iron-steel |
32 | rubber |
33 | heat |
34 | jobs |
35 | lei |
36 | bop |
37 | zinc |
38 | orange |
39 | pet-chem |
40 | dlr |
41 | gas |
42 | silver |
43 | wpi |
44 | hog |
45 | lead |
それ以外のコードはIMDBとほぼ同じです。
OUT_DIR = 'reuters'
# Load data from keras API
(x_train, y_train), (x_test, y_test) = reuters.load_data()
# get word index
word_index = reuters.get_word_index()
# make dictionary to reference index
word_list = {(value+3):key for key,value in word_index.items()}
INVALID_STR = '#$%'
# define invalid string to remove them later
word_list[0] = INVALID_STR
word_list[1] = INVALID_STR
word_list[2] = INVALID_STR
# define class names from ex: https://github.com/keras-team/keras/issues/12072
class_list = ['cocoa','grain','veg-oil','earn','acq','wheat','copper','housing','money-supply',
'coffee','sugar','trade','reserves','ship','cotton','carcass','crude','nat-gas',
'cpi','money-fx','interest','gnp','meal-feed','alum','oilseed','gold','tin',
'strategic-metal','livestock','retail','ipi','iron-steel','rubber','heat','jobs',
'lei','bop','zinc','orange','pet-chem','dlr','gas','silver','wpi','hog','lead']
# make train/test dirs and class dirs
for cid, class_name in enumerate(class_list):
os.makedirs(os.path.join(OUT_DIR,'train', '{:02d}_{}'.format(cid,class_name)), exist_ok=True)
os.makedirs(os.path.join(OUT_DIR,'test', '{:02d}_{}'.format(cid,class_name)), exist_ok=True)
保存するためのフォルダが、
46個できる差はありますが、ほぼ同じです。
# convert train data
for num, (x_data, y_data) in enumerate(zip(x_train, y_train)):
# make file path
fpath = os.path.join(OUT_DIR,'train', '{:02d}_{}'.format(y_data,class_list[y_data]), 'train_{:05d}.txt'.format(num))
with open(fpath, mode='w', encoding='utf-8') as f:
# convert indices and join words with space
word_org = ' '.join(word_list[inx] for inx in x_data )
# remove invalid strings
word_org = word_org.replace(INVALID_STR+' ', '')
# save text
f.write(word_org)
以下のように保存されます。
次回は、
(4) Boston Housing data のcsvへの変換方法
について書きます。
最後まで読んでいただき、ありがとうございます!
ブックマーク登録、
ツイッターフォロー、
よろしくお願いいたします!🙇♂️🙇♂️
↓↓↓